Heterogeneous computer architectures with extensive use of hardware accelerators, such as FPGAs, GPUs, and neural processing units, have shown significant potential to bring in orders of magnitude improvement in compute efficiency for a broad range of applications. However, system designers exploring these non-traditional architectures generally lack effective design methodologies and tools to swiftly navigate through the intricate design trade-offs and achieve rapid design closure. While several heterogeneous computing platforms are becoming commercially available to a wide user base, they are very difficult to program, especially those with reconfigurable logics.
To address these pressing challenges, my research group investigate new applications, programming models, algorithms, and tools to enable highly productive design and implementation of application- and domain-specific computer systems. Our cross-cutting research intersects CAD, machine learning (ML), compiler, and computer architecture. In particular, we are currently tackling the following important and challenging problems:
- Algorithm-Hardware Co-Design for Machine Learning Acceleration
- Multi-Paradigm Programming for Heterogeneous Computing
- High-Level Synthesis of Fast and Secure Accelerators
- Learning-Assisted IC Design Closure
My group is investigating various accelerator architectures for compute-intensive ML applications, where we employ an algorithm-hardware co-design approach to achieving both high performance and low energy. Specifically, we are exploring new layer types and quantization techniques in deep neural networks that map very efficiently to FPGA/ASIC accelerators. Examples include channel gating [C58][C59], precision gating [C61], unitary group convolution [C57], and outlier channel splitting [C56]. We are also among the first to design and implement a highly efficient binarized neural network (BNN) accelerator, which is demonstrated on FPGAs [C35][W3], and included as part of the 16nm 385M-transistor Celerity SoC (opencelerity.org) [C39][W4][J8]. In addition, we are developing a new suite of realistic benchmarks for software-defined FPGA-based computing [C42]. Unlike previous efforts, we aim to provide parametrizable benchmarks beyond the simple kernel level by incorporating real applications from emerging domains.
The latest advances in industry have produced highly integrated heterogeneous hardware platforms, such as the CPU+FPGA multi-chip packages by Intel and the GPU and FPGA enabled AWS cloud by Amazon. Although these heterogeneous computing platforms are becoming commercially available to a wide user base, they are very difficult to program, especially with FPGAs. As a result, the use of such platforms has been limited to a small subset of programmers with specialized knowledge on the low-level hardware details.
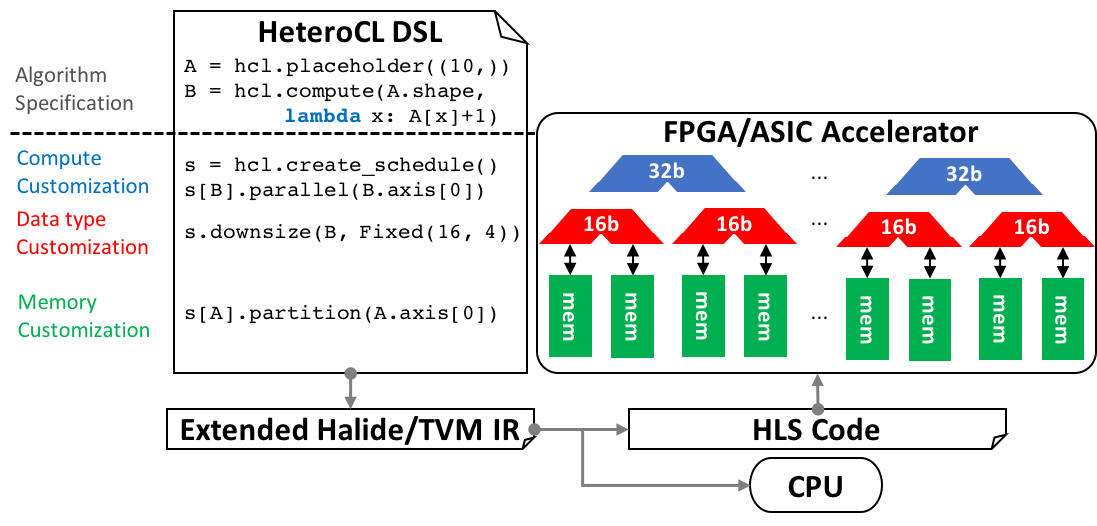
To democratize accelerator programming, we are developing HeteroCL, a highly productive multi-paradigm programming infrastructure that explicitly embraces heterogeneity to integrate a variety of programming models into a single, unified programming interface [C47]. The HeteroCL DSL provides a clean programming abstraction that decouples algorithm specification from three important types of hardwarecustomization in compute, data types, and memory architectures. It further captures the interdependence among these different customization techniques, allowing programmers to explorevarious performance/area/accuracy trade-offs in a systematic andproductive manner.
Specialized hardware manually created by traditional register-transfer-level (RTL) design can yield high performance but is also usually the least productive. As specialized accelerators become more integral to achieving the performance and energy goals of future hardware, there is a crucial need for above-RTL design automation to enable productive modeling, rapid exploration, and automatic generation of customized hardware based on high-level languages. Along this line, there has been an increasing use of high-level synthesis (HLS) tools to compile algorithmic descriptions (e.g., C/C++, Python) to RTL designs for quick ASIC or FPGA implementation of hardware accelerators [J5][J4].
While the latest HLS tools have made encouraging progress with much improved quality-of-results (QoR), they still heavily rely on designers to manually restructure source code and insert vendor-specific directives to guide the synthesis tool to explore a vast and complex solution space. The lack of guarantee on meeting QoR goals out-of-the-box presents a major barrier to non-expert users. Moreover, the security aspects of the synthesized accelerators have been largely overlooked so far. To this end, we are developing a new generation of HLS techniques that feature scalable cross-layer synthesis [C25][C26] and exact optimization [C43], complexity-effective dynamic scheduling [C28][J7][C33], trace-based analysis [C36], and information flow enforcement [C46] to enable a greatly simplified hardware design experience, while achieving a high performance and satisfying the security constraints.
A primary barrier to rapid hardware specialization is the lack of guarantee by existing CAD tools on achieving design closure in an out-of-the-box fashion. Mostly, using these tools requires extensive manual effort to calibrate and configure a large set of design parameters and tool options to achieve a high QoR. Since CAD tools are at least two order of magnitude slower than a software compiler, evaluating just a few design points could already be painfully time-consuming, as physical design steps such as place-and-route (PnR) commonly consume hours to days for large circuits.
We believe there is a great potential for employing ML across different stages of the design automation stack to expedite design closure by (1) minimizing human supervision in the overall design tuning process and (2) significantly reducing the time required to obtain accurate QoR estimation for a given design point. Along these directions, we have demonstrated the efficacy of our approach by applying ML to distributed bandit-based autotuning for FPGA timing closure [C34][C48], low-error resource estimation in HLS [C44], and fast yet highly accurate power inference for resusable ASIC IPs [C54].
Research conducted by my group has been sponsored by Defense Advanced Research Projects Agency (DARPA), National Science Foundation (NSF), Semiconductor Research Corporation (SRC), Facebook (now Meta), Google, Intel, Microsoft Azure, Qualcomm, SambaNova Systems, and Xilinx (now AMD). Their support is greatly appreciated.